-
Notifications
You must be signed in to change notification settings - Fork 120
New issue
Have a question about this project? Sign up for a free GitHub account to open an issue and contact its maintainers and the community.
By clicking “Sign up for GitHub”, you agree to our terms of service and privacy statement. We’ll occasionally send you account related emails.
Already on GitHub? Sign in to your account
why sum weights? #15
Comments
The construct above until the highlighted line is for selecting the weight per each label. For example, if your target label is (one-hot-encoded) class 1, you would use those lines to select the weight corresponding to that index. One could simplify the lines weights = torch.tensor(weights).float()
weights = weights.unsqueeze(0)
weights = weights.repeat(labels_one_hot.shape[0],1) * labels_one_hot
weights = weights.sum(1) by simply saying weights = weights[labels_one_hot.argmax(1)] # pick the correct weight for each label
# weights = weights[labels.long()] # this would also do Here's a snippet you can use to verify: # gen dummy labels
num_classes = 5
labels = torch.randint(num_classes, (100, ))
labels_one_hot = torch.eye(num_classes)[labels]
# gen dummy weights
weights = torch.randn(num_classes)
# original method
weights0 = weights.unsqueeze(0)
weights0 = weights0.repeat(labels_one_hot.shape[0],1) * labels_one_hot
weights0 = weights0.sum(1)
# method 1
weights1 = weights[labels_one_hot.argmax(1)]
#
weights2 = weights[labels.long()]
print(torch.equal(weights0, weights1), torch.equal(weights0, weights2))
>> True True |
Sign up for free
to join this conversation on GitHub.
Already have an account?
Sign in to comment
why add this sum make all class weight the same
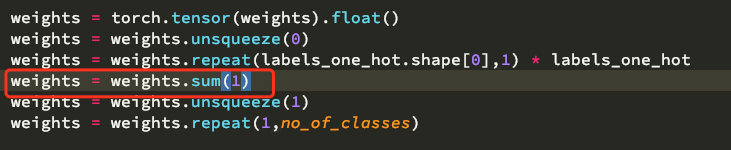
The text was updated successfully, but these errors were encountered: